An Outer Space View of Business Cycles
- Business Cycle
- KOF Bulletin
- World Economy
Nighttime lights data captured by satellites can provide useful information regarding the current economic activity across countries and regions, and also for the prediction of future developments. In a recently published KOF Working Paper, Jaqueson K. Galimberti presents his latest research and promising results favouring the use of this innovative source of data for macroeconomic measurement and forecasting.
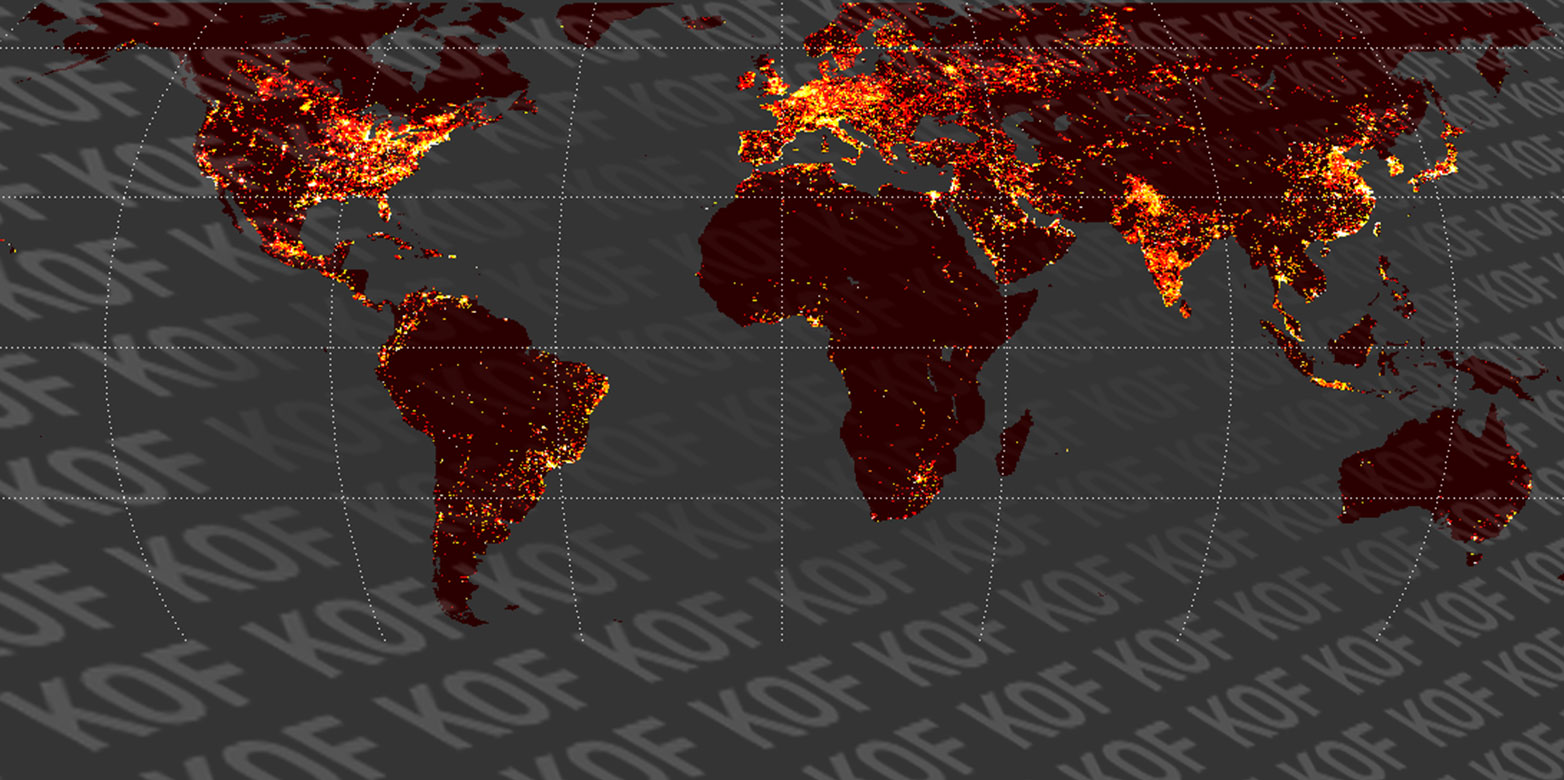
Forecasts of economic activity are crucial to the decision-making process of policymakers and market participants in general. In order to improve the accuracy of model-based forecasts, applied research tends to focus on finding and evaluating new data sources and on developing methods to extract and combine predictive information from these data. Traditional sources of data for macroeconomic forecasting include so-called hard data, produced by statistical agencies to reflect direct measurements of economic activity; soft data, collected from market participants in the form of surveys; and financial data, often providing high frequency information about market perceptions. A relatively newer source of data that has received growing attention in economic literature is data provided by nighttime lights observations, captured by optical sensors onboard satellites orbiting Earth. In his Paper, Jaqueson K. Galimberti proposes an evaluation of the usefulness of nightlights data for the prediction of annual GDP growth across a global sample of 172 countries.
Nightlights data provide accurate measurements of human activity on the ground
The underlying hypothesis in the use of nightlights to predict a country’s GDP is that the emission of lights indicate the presence of economic activity in that country’s territory. For forecasting purposes it is the dynamics of such a relationship that matter. In other words, the usefulness of the nightlights data for macroeconomic forecasting depends on whether lagged light measurements provide leading signals of current and future economic activity. In this context, perhaps the greatest advantage of nightlights data over traditional sources of macroeconomic data is that they allow a more precise geographical mapping of economic activity through timely snapshots of what is happening in a given region over a given period. Hence, the nightlights data are less prone to the type of measurement errors affecting national accounting statistics, often leading to data revisions, and they can provide location-based predictive signals of aggregate economic activity, for example by capturing the geographical spread of production chains across regions.
Richness of nightlights information allows construction of several indicators
Thus, for forecasting purposes, current observations of lights require processing into the form of leading indicators of future economic activity, and there are several possibilities along this dimension. In fact, the main contribution of Galimberti’s paper is its proposal of innovative measures for the extraction of predictive signals of macroeconomic activity from the richness of information provided by the nightlights dataset. Such indicators are classified into three categories: (i) aggregate indicators, (ii) distribution-based indicators and (iii) location-based indicators. The paper provides further details about these indicators. Graph G 1 depicts an illustration of the distribution-based indicators for the case of Spain in 2013.
Nightlights indicators find useful application across a global sample of countries
Processing the sample of available nightlights data yields the time series input for the construction of model-based forecasts of annual GDP growth for 172 countries over the period 1993-2014. These forecasts are constructed by augmenting a benchmark first-order autoregressive model on GDP growth rates with the lagged values of the nightlights indicators. Compared to the benchmark specification, the evidence tends to be favourable to the incorporation of nightlights data for GDP growth forecasting: in-sample accuracy improvements, averaged across countries using their GDP levels as weights, range from 2.9 per cent to 7.2 per cent, depending on the indicator.
The results also point to some heterogeneity of performance across countries. Graph 2 illustrates this finding for a selection of countries, showing how the average improvements can conceal substantial variability of individual performances. Interestingly, these performances do not seem to be related to the countries levels of development. In out-of-sample tests, i.e. restricting the use of data for model estimation to that available to a forecaster at the time the forecast is made, the performance of the nightlights-based forecasts deteriorate, a result mainly caused by the unavailability of large enough samples of data at the country-individual level. Panel data specifications seem to provide an interesting alternative in the latter circumstances, although the data provides weak support to the assumption of a common relationship between the nightlights and GDP across countries.
Jaqueson K. Galimberti: Forecasting GDP growth from the outer space. KOF Working Papers, (2017) Zürich: KOF, ETH Zürich.
Contact
No database information available