New Technologies and Productivity: What Role Does the Educational Level Play?
- Innovation
- KOF Bulletin
- Education
There are frequent reports in the news suggesting that information and communication technologies lead to higher productivity especially for highly educated workers. However, most of the studies consider only the complementary effect of new technologies and tertiary educated workers. A new study investigates the effect these technologies have on workers with different levels of education.
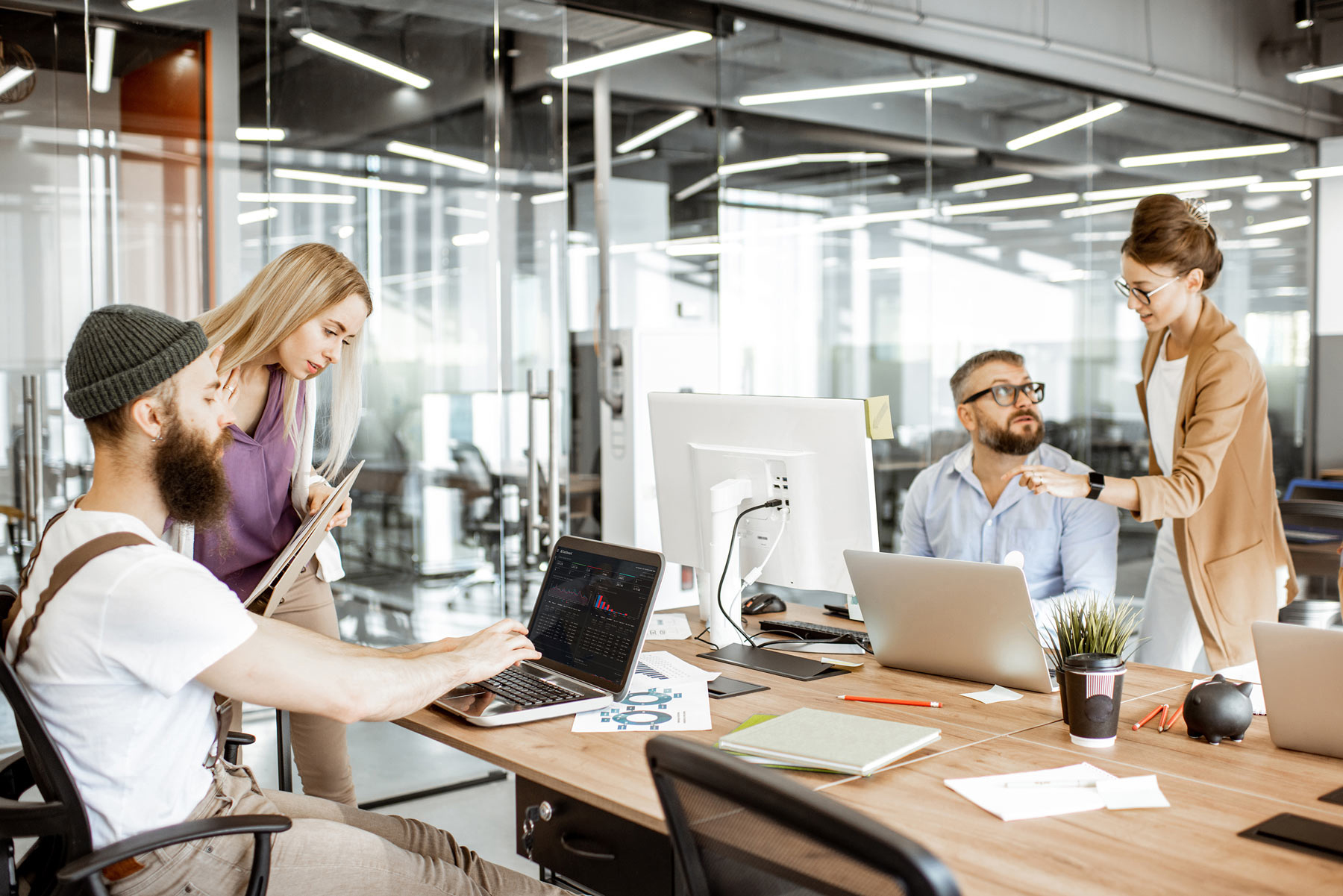
The economic literature largely suggests that new technologies lead to higher productivity, especially for highly educated workers, who possess the necessary skills to use them. Yet despite the complexity of information and communication technologies (ICT), most of this research mainly views ICT as a homogeneous input factor when evaluating its impacts on firms' productivity. However, viewing ICT as a unique measure of technological capital is imprecise, because information technologies (IT) and communication technologies (CT) have different effects on productivity.
Differences between IT and CT
IT makes accessing information less expensive, thereby giving workers more autonomy and a wider span of control. IT thus acts as a decentralizing force that allows workers to handle situations more autonomously. Therefore, IT increases the productivity of workers in the middle of the education distribution, who use IT for the production process, while workers at the lower and upper end of the education distribution profit relatively less from the effect of IT. Examples of IT are business management tools such as Enterprise Resource Planning (ERP), Supply Chain Management (SCM), or Customer Relationship Management (CRM).
In contrast, CT reduces communication costs and therefore leads to more centralized management. CT thus acts as a centralizing force that shifts decision-making responsibilities from the production level to the management level. Therefore, highly educated workers, who frequently hold managerial positions, profit more from the use of CT. Conversely, CT has almost no effect on productivity for poorly educated workers. A prime example of CT is the use of intranet among employees.
This analysis relies on a firm-level survey conducted by the KOF Swiss Economic Institute between 2005 and 2017. The survey includes information on both workers’ education level and the use of technologies. Workers are subdivided into four groups according to their highest level of education: workers with no post-compulsory education (“Lower”), workers with upper-secondary vocational education (“Trained”), workers with a tertiary vocational education (“Advanced”), and workers with a tertiary academic education (“Academic”). CT is measured as the share of workers who regularly use the intranet. IT is measured as firms' use of ERP, CRM, and SCM.
IT particularly beneficial for workers with a tertiary vocational education
Graph G11 presents the measure of complementarity between IT and the four educational groups. The measure is highest for Advanced workers and IT. Consequently, this is where the greatest complementarity exists. In other words, the availability of IT increases the productivity of Advanced workers the most. The complementarity of IT and Trained as well as IT and Academic workers is less high, while the complementarity of IT and Lower workers is lowest.
CT especially complementary to Academic
Graph G12 presents the measure of complementarity between CT and the four educational groups. This figure suggests that CT is especially complementary with Academic workers. All other measures show a similarly high degree of complementarity with IT.
The study shows that the degree of complementarity between ICT and workers' education is highly heterogeneous across technologies. For IT use, the estimations show that workers with a tertiary vocational education exhibit the largest complementarity. In contrast, workers with a tertiary academic education exhibit the largest degree of complementarity with CT.
Empirical methods
To assess complementarities, the authors use quantitative regression analysis. Specifically, they estimate firm-level production functions, including measures of workers' level of education; use of IT or CT; and interaction terms between these two groups of inputs. The relative size of the interaction terms between the educational variables and these technologies represents the degree of complementarity. The 95% confidence intervals show the statistical uncertainty. The panel structure of the data account for unobserved heterogeneity by exploiting within-firm variation over time. The estimations further control for physical capital and for computer user share. Controlling for computer user share allows to account for unobserved omitted variable bias due to other computer-based technologies.
Contacts
No database information available
No database information available