Forecasting yesterday, today and tomorrow
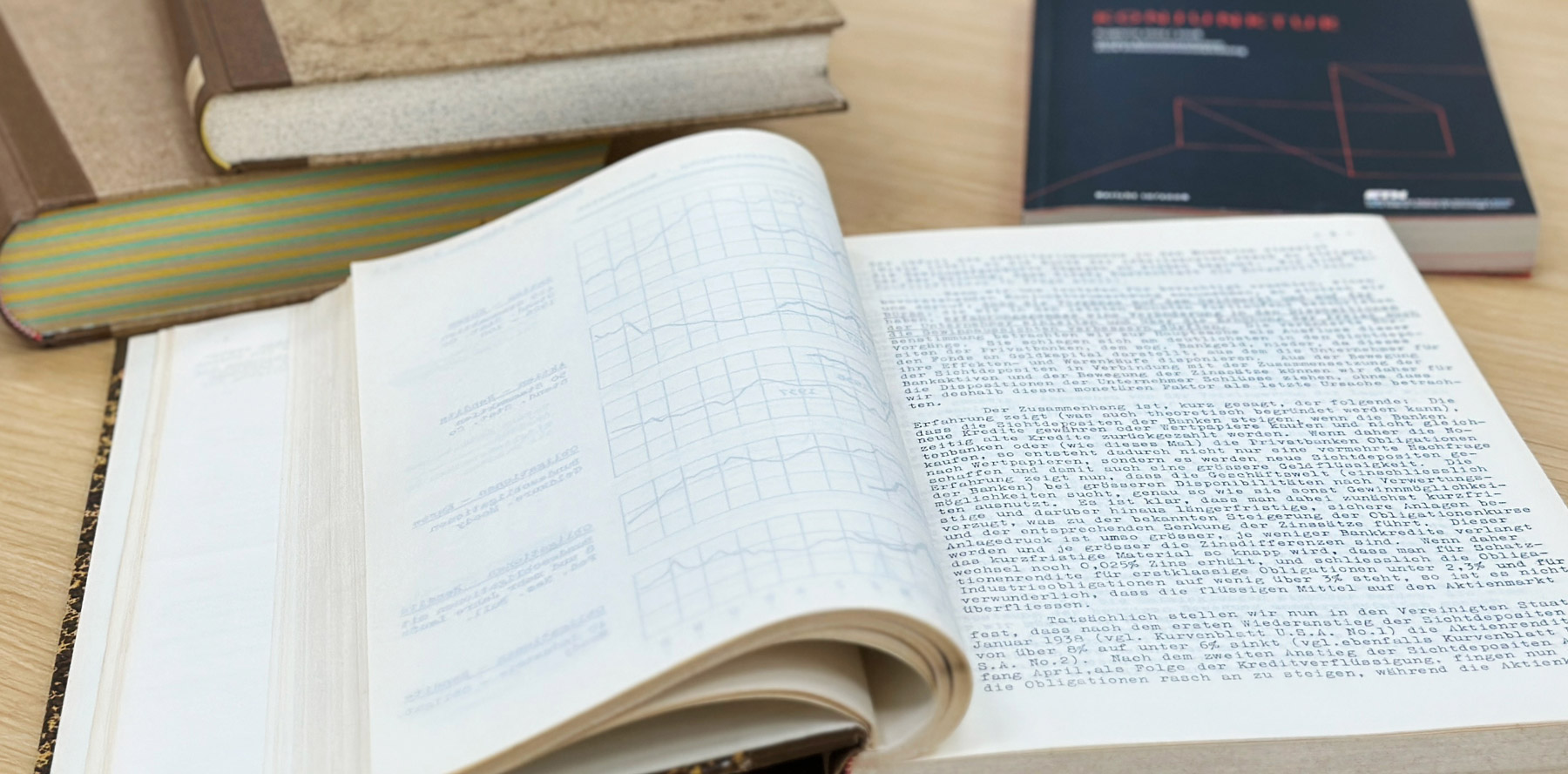
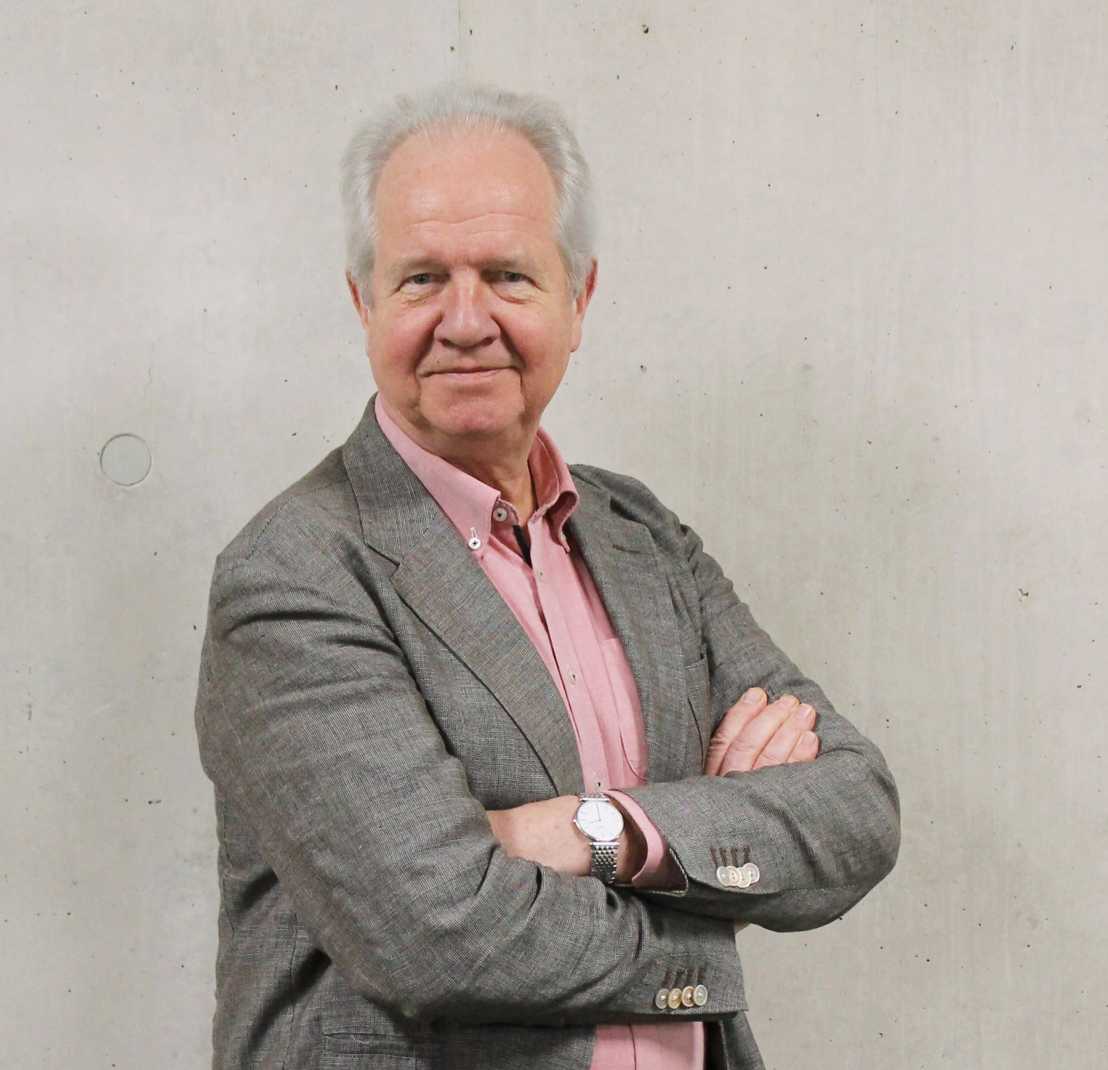
When the young econometrician Yngve Abrahamsen – now 66 years old – produced his first economic models for Switzerland at the University of St Gallen (HSG) in the early 1980s, punch cards had only recently disappeared from the workplace. He had to wait the entire night for the mainframe computer in Zurich to output its calculations.
Abrahamsen moved to KOF in 1996 and saw first-hand how, over the course of his long career at ETH Zurich, the methods used to calculate economic forecasts were continually refined – partly thanks to increasing computing power.
“We should not blindly trust models.”Yngve Abrahamsen
Macroeconomic models had actually been used since as far back as 1974 to produce forecasts of the Swiss economy. But whereas expertise, intuition, experience, economic policy convictions and gut instinct still played a key role as part of the heuristic methods used in the early years, the model approach became increasingly driven by modern modelling techniques and statistical methods over the years and decades. The aim was to establish the perception of KOF as an empirical economic research institute that was independent of any vested interests.
That does not mean, however, that models can now replace humans, as every model has its limitations. “We should not blindly trust models”, says Abrahamsen. Human expertise, he points out, is essential – even in the age of artificial intelligence (AI). Common sense helps to exclude spurious correlations, according to Abrahamsen, who was closely involved in the development and refinement of KOF’s so-called ‘macro-model’ in his capacity as head of the Swiss Macroeconomic Forecasting section for more than two decades from 2002 until his retirement in 2023. A total of 635 variables were most recently input into KOF’s macro-model. 328 variables were calculated for the Swiss and international economies, 50 of them using stochastic equations.
The buzzword now is ‘model pluralism’
Nowadays, several models are used simultaneously to produce quarterly economic forecasts. A change of generations – Dr Samad Sarferaz (45 years old) and Dr Alexander Rathke (46 years old) have borne the main responsibility for forecasting since 2023 – has brought with it a new generation of models.
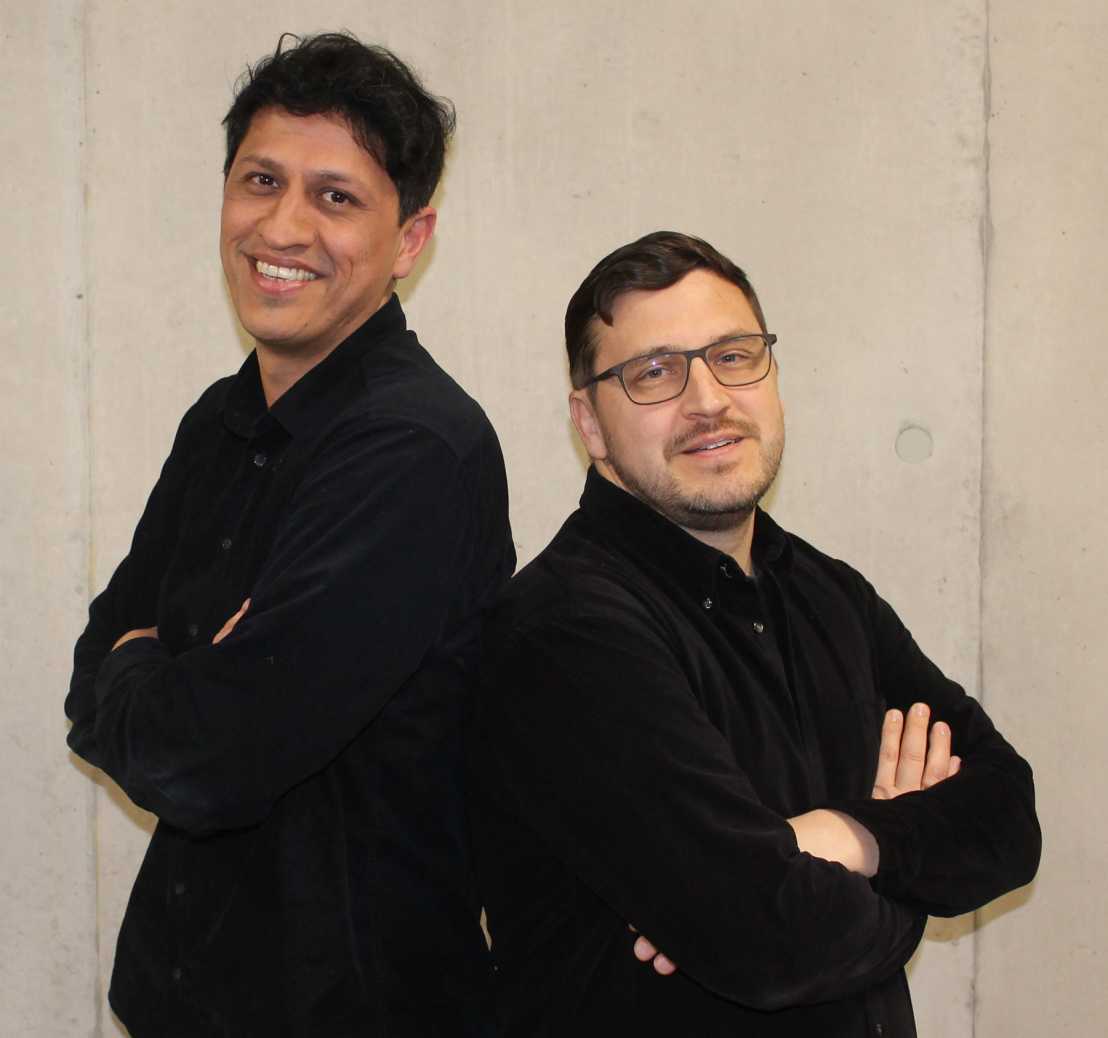
“We like to talk of ‘model diversity’ or ‘model pluralism’, explains Samad Sarferaz, co-head of the Macroeconomic Forecasting and Data Science research division. Four or five models run simultaneously during the forecasting process. “Each of them has its own strengths and weaknesses”, adds Sarferaz. One forecasting model – Bayesian VAR – was specially adapted and implemented by him and Alexander Rathke to be used at KOF and learns, for example, from its own forecasting errors. However, he explains, these learning processes are often opaque to researchers. Everything is somehow dependent on everything else. “It’s almost like a black box”, says Sarferaz.
“The models are appropriate to varying degrees, depending on what we want to forecast. As in any toolbox, not every screwdriver is suitable for every screw.”Alexander Rathke
When classic macro-models are used, economic theory plays a much bigger role, there is a clearly defined relationship between variables, and researchers know where their results come from. However, these models are limited in their ability to learn from data and do not possess the flexibility of modern, data-driven approaches.
Human, model and machine
“The models are appropriate to varying degrees, depending on what we want to forecast. As in any toolbox, not every screwdriver is suitable for every screw”, explains Alexander Rathke, head of the Swiss Macroeconomic Forecasting section since last year. Like his predecessor Yngve Abrahamsen, Rathke also believes that humans are an irreplaceable factor. “It may happen that, owing to one-off factors, certain knowledge is not included in the model. The technical experts can then fine-tune that”, he adds. Moreover, an economic forecast relies not only on a numerical value but also on a consistent backstory, according to Rathke, and humans are still much better at this kind of interpretation than machines are.
KOF combines the factors ‘human, model and machine’ as part of a lengthy, sophisticated process. “In Switzerland this is unique in terms of its depth and breadth”, explains Rathke. Approximately 20 experts are involved in producing such a forecast.
“Forecasts provide guidance and are comparable to a weather report. Even if they are not always 100 per cent accurate, they are useful for planning purpose.”Samad Sarferaz
The KOMA macro-model recently developed at KOF enables not only precise economic forecasts to be made but also improves the representation of uncertainty, adds Sarferaz. “The model’s output also immediately produces an uncertainty calculation. Now it no longer needs to be computed retrospectively.” This can convey to readers of the economic report an impression of the forecast’s accuracy.
Empiricism rather than ideology
Totally precise forecasting is the exception rather than the rule and is not the objective anyway, according to Sarferaz. “Forecasts provide guidance and are comparable to a weather report. Even if they are not always 100 per cent accurate, they are useful for planning purposes”, he explains.
“Data quality is more important than data quantity.”Yngve Abrahamsen
Like Abrahamsen, Sarferaz also believes it is an advantage that the data-based model approach largely conceals any economic policy views. “Our thinking is empirically driven. We do not belong to any ideological group. We have to be philosophically flexible in order to learn from the data.”
Signal versus noise
However, it is not always necessarily better to have more data. “We always have to ask ourselves how much signal and how much noise additional data create. If they only create more noise, additional data do more harm than good”, explains Rathke. Abrahamsen agrees. “Data quality is more important than data quantity”.
Asked about his hobby, the passionate sailor explains what this sport shares in common with economic forecasting. “When sailing, you always have to consider environmental conditions such as the weather. Only if you analyse the data thoroughly can you navigate the boat safely.” He believes that economic forecasting is similar. “Good forecasters need to keep up to speed both in terms of data and methods as well as with respect to political and economic policy debates”, adds Abrahamsen.
“Every economic crisis and every economic scenario has its particular characteristics and is not exactly the same as in the past. ”Jan-Egbert Sturm
Large language models and big data: proprietary AI tools as a vision for the future
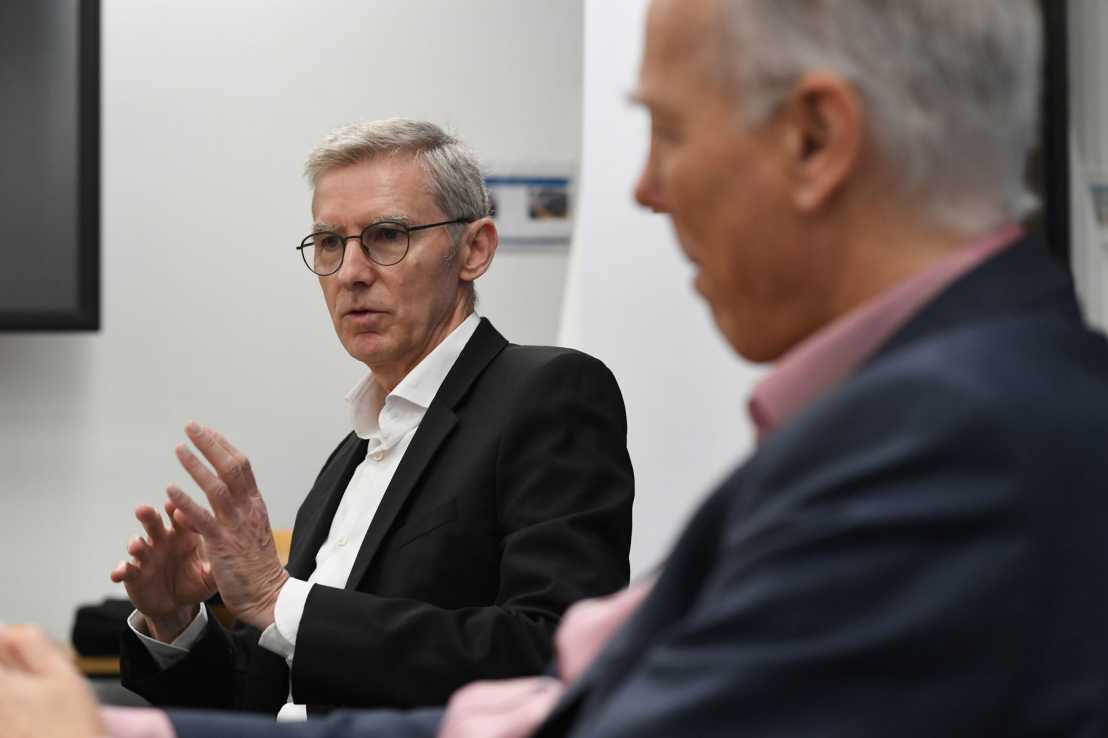
And what does the future hold for KOF’s economic forecasting? Jan-Egbert Sturm and Hans Gersbach, KOF’s two directors, have a clear vision in this respect. “We hope that the growing use of artificial intelligence to analyse data, to carry out programming and to write texts will enable us to produce economic forecasts even better and more quickly”, they say – before making an important caveat. “It would be wrong to automate the use of AI. We still need to verify its output”, explains Hans Gersbach.
“We hope that the growing use of artificial intelligence [...] will enable us to produce economic forecasts even better [...]. At the heart of this project are so-called large language models, which function like neural networks and can be trained using huge amounts of data so they can help us analyse economic scenarios.”Hans Gersbach
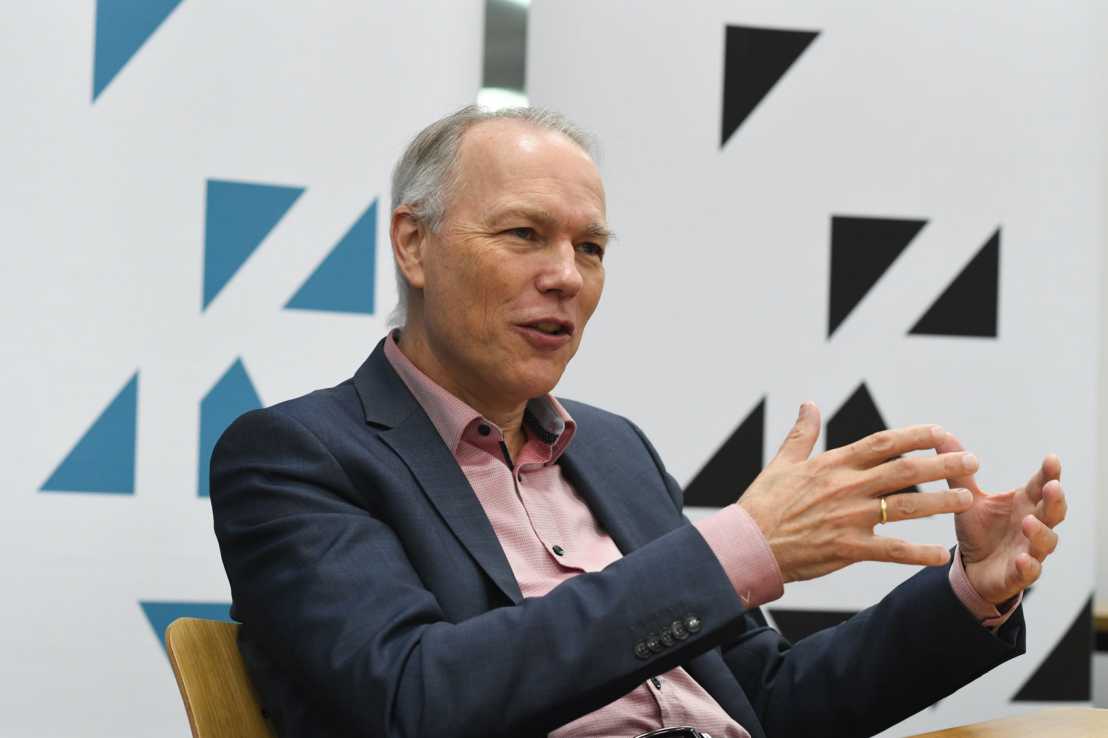
“Every economic crisis and every economic scenario has its particular characteristics and is not exactly the same as in the past. In such situations it is therefore not possible purely to use data from the past; you also have to draw on human expertise so that you include knowledge that is not yet contained in the data”, adds Jan-Egbert Sturm. He sees it as a strength of KOF’s forecasting that several rounds of discussions yield expertise that the computer world is still unable to deliver. During the COVID-19 pandemic, for example, previously tried-and-tested models suddenly did not function properly any more, so KOF had to rely more on scenarios and experts at this time.
KOF plans to use artificial intelligence to develop its own tools in future. It has already launched its first project in collaboration with the AI Center at ETH Zurich. “At the heart of this project are so-called large language models, which function like neural networks and can be trained using huge amounts of data so they can help us analyse economic scenarios”, explains Hans Gersbach.